Impact of Using Poor Quality Data and Metrics to Measure Data Quality
In the previous article, we have understood why data quality is important in the field of risk management. We also had a closer look at the various characteristics which constitute data quality as well as the processes that need to be put in place in order to manage data quality.
In this article, we will go a couple of steps further. First, we will try to understand what the impacts of using poor quality data are. Secondly, we will also try to understand how organizations build data quality metrics that help them ensure the consistency of data across various processes on a daily basis.
Impact of Using Poor Quality Data
If proper data quality management is not set up in order to complement the risk management system, then the impact can be huge. The various types of impact which may be faced by an organization have been listed in this article.
- Financial Impact: The most obvious impact of poor quality data would show in the form of financial impact. This is because poor quality data used as input for risk management models will lead to poor quality business decisions.
The management and stakeholders will not be aware of the type of risk that they are undertaking. They will continuously be underestimating risk and making riskier bets than they should be making. This can have an adverse impact on the short-term and long-term finances of the firm. It can also jeopardize the survival of the company over the long run.
- Regulatory Impact: Financial institutions have to abide by the rules implemented by many regulatory bodies. This means that they need to have systems in place to quickly collate their data and then share it with regulatory bodies at regular intervals of time.
Organizations are supposed to ensure that their risk metrics stay within a certain threshold failing which they have to pay a monetary penalty. Hence, if data quality is not managed properly and poor data is shared with regulatory bodies, there could be financial loss in the form of penalties along with reputation loss as well.
- Confidence Impact: If the data quality used in a risk management system is not consistent, it could cause confidence issues amongst managers. These issues could be related to both overconfidence as well as lack of confidence.
For instance, managers may wrongly assume that they have thoroughly studied all the risks and hence are prepared for the worst possible outcome whereas in reality that may not be the case.
Hence, faulty data may give false confidence which could ultimately lead to bad decisions and financial losses. On the other hand, it is also possible that the managers may not have any confidence in the results given by the risk management system. In such cases, they may not be able to take calculated risks and hence take full advantage of the risk management system.
- Productivity Impact: The absence of good quality data can be frustrating for employees in the risk management department. This is because it increases their re-work. The model may not give the correct output and hence they may have to collate data again and re-run the model. This leads to delays in processing time which can be time-consuming.
Delays can be particularly dangerous since managing market risks is all about timing. If the decision is not taken at the correct time, then the impact can be humungous since security prices can fluctuate wildly in a matter of a few hours.
Metrics to Measure Data Quality
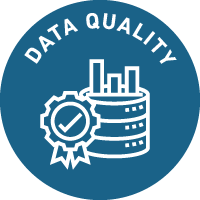
In order to manage the data quality across different time periods, organizations need to find a way to quantify this data quality. This is done by using data quality metrics. Data quality metrics can be of two types. The difference between the two types has been explained below:
- A base-level data quality metric is easily quantifiable. It checks features such as completeness and accuracy and can be easily expressed in the form of numbers. However, it has limited applicability meaning that it cannot be used to gauge the quality level of all data types.
- A complex data quality metric considers the financial impact of poor quality data at different parts of the organizational process. This type of metric allows the organization to discover exactly where the incorrect data was generated and hence what measures can be taken to fix it. The problem with this metric is that it is difficult to compute and such a system is often expensive to deploy and maintain.
Hence, the bottom line is that data quality issues can be very expensive. This is because they are the raw material for several processes in the risk management domain. If the raw material itself is of poor quality, then so is the outcome.
❮❮ Previous | Next ❯❯ |
Related Articles
Authorship/Referencing - About the Author(s)
![]()
The article is Written and Reviewed by Management Study Guide Content Team. MSG Content Team comprises experienced Faculty Member, Professionals and Subject Matter Experts. We are a ISO 2001:2015 Certified Education Provider. To Know more, click on About Us. The use of this material is free for learning and education purpose. Please reference authorship of content used, including link(s) to ManagementStudyGuide.com and the content page url.